bwin必赢系列讲座名家讲坛第2期——Large Neural Models' Self-Learning Symbolic Knowledge
报告题目(Title):Large Neural Models' Self-Learning Symbolic Knowledge
时间(Date & Time):2023.7.5 10:00-12:00
地点(Location):理科一号楼1453(燕园校区) Room 1453, Science Building #1 (Yanyuan)
主讲人(Speaker):Heng Ji
邀请人(Host):Sujian Li
报告摘要(Abstract):
Recent large neural models have shown impressive performance on various data modalities, including natural language, vision, programming language and molecules. However, they still have surprising deficiency (near-random performance) in acquiring certain types of knowledge such as structured knowledge and action knowledge. In this talk I propose a two-way knowledge acquisition framework to make symbolic and neural learning approaches mutually enhance each other. In the first stage, we will elicit and acquire explicit symbolic knowledge from large neural models. In the second stage, we will leverage the acquired symbolic knowledge to augment and enhance these large models.
I will present three recent case studies to demonstrate this framework:
(1) The first task is to induce event schemas (stereotypical structures of events and their connections) from large language models by incremental prompting and verification [Li et al., ACL2023], and apply the induced schemas to enhance event extraction and event prediction.
(2) In the second task, we noticed that current large video-language models rely on object recognition abilities as a shortcut for action understanding. We utilize a Knowledge Patcher network to elicit new action knowledge from the current models and a Knowledge Fuser component to integrate the Patcher into frozen video-language models.
(3) In the third task, we use large-scale molecule-language models to discover molecule subgraph structures ("building blocks") which contribute to blood brain barrier permeability in the kinase inhibitor family, and propose several candidate kinase inhibitor variants with improved ability to pass the blood brain barrier to accelerate better drug discovery. Then we can encode such graph pattern knowledge using lightweight adapter modules, bottleneck feed-forward networks that are inserted into different locations of backbone large molecule-language models.
主讲人简介(Bio):

Heng Ji is a professor at Computer Science Department, and an affiliated faculty member at Electrical and Computer Engineering Department and Coordinated Science Laboratory of University of Illinois Urbana-Champaign. She is an Amazon Scholar. She is the Director of Amazon-Illinois Center on AI for Interactive Conversational Experiences (AICE). She received her B.A. and M. A. in Computational Linguistics from Tsinghua University, and her M.S. and Ph.D. in Computer Science from New York University. Her research interests focus on Natural Language Processing, especially on Multimedia Multilingual Information Extraction, Knowledge-enhanced Large Language Models, Knowledge-driven Generation and Conversational AI. She was selected as "Young Scientist" and a member of the Global Future Council on the Future of Computing by the World Economic Forum in 2016 and 2017. She was named as part of Women Leaders of Conversational AI (Class of 2023) by Project Voice. The awards she received include "AI's 10 to Watch" Award by IEEE Intelligent Systems in 2013, NSF CAREER award in 2009, PACLIC2012 Best paper runner-up, "Best of ICDM2013" paper award, "Best of SDM2013" paper award, ACL2018 Best Demo paper nomination, ACL2020 Best Demo Paper Award, NAACL2021 Best Demo Paper Award, Google Research Award in 2009 and 2014, IBM Watson Faculty Award in 2012 and 2014 and Bosch Research Award in 2014-2018. She was the associate editor for IEEE/ACM Transaction on Audio, Speech, and Language Processing, and served as the Program Committee Co-Chair of many conferences including NAACL-HLT2018 and AACL-IJCNLP2022. She is elected as the North American Chapter of the Association for Computational Linguistics (NAACL) secretary 2020-2023.
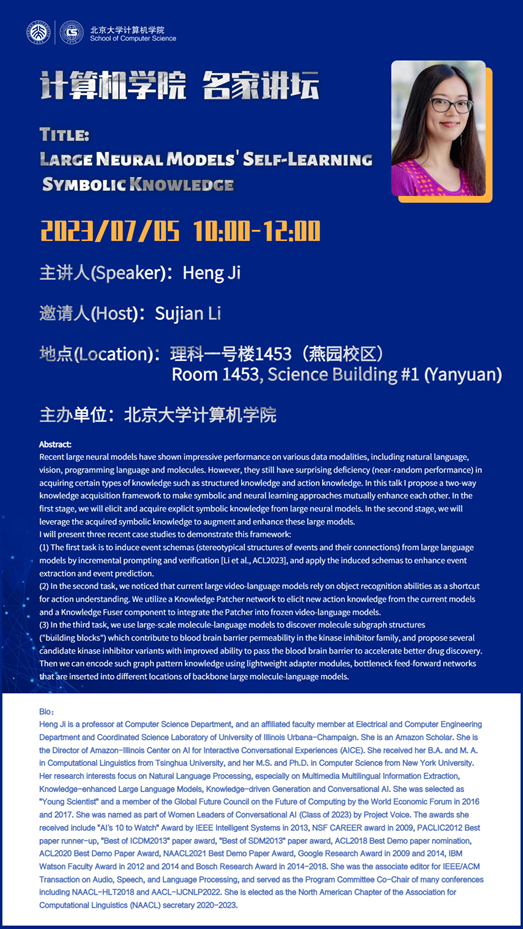
欢迎关注bwin必赢微信公众号,了解更多讲座信息!
bwin必赢
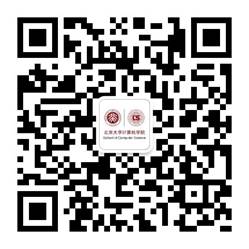